What are some specific AI technologies? And some examples of successful AI
In a previous article, we mentioned synthetic generation, its myths, applications, and its significance in modern-day and destiny society. today we can speak about the particular technologies utilized in AI.
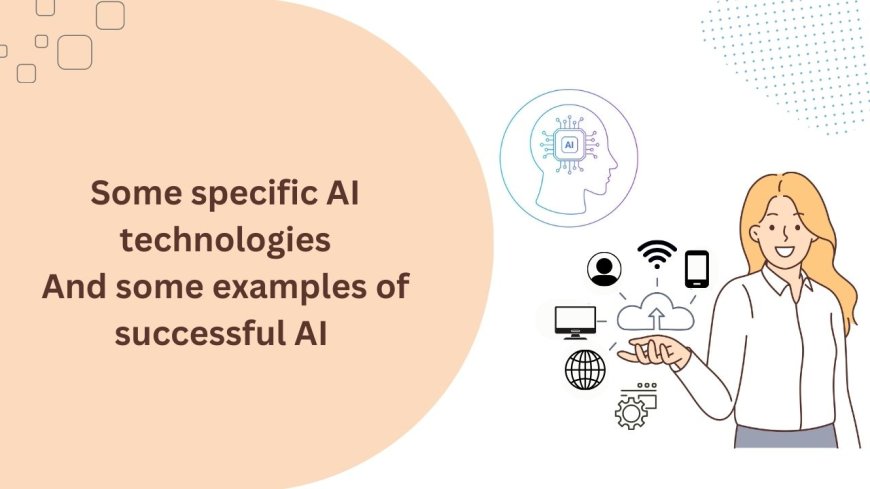
Some specific AI technologies mentioned in the provided sources include:0
Natural Language Generation (NLG):
NLG is used for producing text from computer data and is currently applied in customer service, report generation, and summarizing business intelligence insights.
Natural Language Generation (NLG) is a branch of Artificial Intelligence that focuses on automatically generating human-like language from data. NLG systems take computerized data and convert it into natural language representation, producing text based on collected data and user input. NLG involves generating text that is logical, contextually appropriate and sounds like human speech, aiming to create reports, summaries, or content readable by humans.
NLG works by converting structured data into natural language text, focusing on creating human language rather than understanding it as Natural Language Processing (NLP) does. NLG tasks include text summarization, question answering, report generation, and dialogue generation.
NLG systems use techniques like content planning, sentence planning, and sentence realization to ensure grammatically correct, contextually relevant, and coherent text output
Speech Recognition:
This technology transcribes and transforms human speech into a format useful for computer applications, commonly used in interactive voice response systems and mobile applications.
Speech recognition, also known as automatic speech recognition (ASR) or speech-to-text, is a technology that enables computers and applications to understand human speech and convert it into written text. It involves the conversion of spoken language into readable text through the use of artificial intelligence algorithms, particularly in deep learning and neural networks.
Speech recognition systems work by analyzing voice modulation, accent, speed of words, voice pitch, and other factors to understand spoken commands or queries. They use techniques like CTC (Connectionist Temporal Classification), transfer learning, pretraining, streaming speech recognition, noise robustness, and multilingual support to enhance accuracy, reduce latency, improve performance in noisy environments, and support multiple languages effectively.
Virtual Agents:
Virtual agents, ranging from simple chatbots to advanced systems, are used in customer service, and support, and as smart home managers, facilitating interactions with humans.
Virtual agents, also known as intelligent virtual agents (IVAs) or virtual assistants, are software programs that leverage artificial intelligence (AI) and natural language processing (NLP) to provide automated service, guidance, or support to users. These virtual agents can interact with users through chat interfaces, emails, or live chat on websites, handling routine customer queries, fulfilling standard requests, and addressing simple problems without the need for human intervention.
Virtual agents are designed to understand user intents, learn from interactions, and execute actions to meet user requests, continuously improving their ability to interpret and respond to user input. They go beyond basic chatbots by not only responding but also understanding, learning, and performing tasks to assist users effectively.
Virtual agents can automate actions that users would typically do themselves, such as sending a text, searching for information, or playing a song, making them valuable tools for businesses looking to optimize customer interactions and streamline processes.
Virtual agents are sophisticated AI-driven tools that enhance customer service operations, automate repetitive tasks, improve user experiences, and offer businesses a scalable and cost-effective solution to handle customer interactions effectively.
Machine Learning Platforms:
These platforms provide algorithms, APIs, development and training toolkits, data, and computing power to design, train, and deploy models into applications, primarily used in enterprise applications for prediction or classification tasks.
The search results provide comprehensive information about machine learning platforms, highlighting their importance, benefits, features, and popular examples in the industry. Machine learning platforms are specialized software solutions that enable users to manage data preparation, machine learning model development, deployment, and monitoring in a unified ecosystem.
These platforms automate data workflows, accelerate data processing, optimize functionality, and facilitate the development, deployment, and improvement of machine learning algorithms.
Some essential features of machine learning platforms include data ingestion, data preparation, feature engineering, model training, model deployment, model management, model comparison, accessible development environments, support for familiar frameworks, enterprise-grade governance, security, and control. These platforms enable users to manage the entire data lifecycle, from data ingestion to inference, and provide tools for tracking metrics, comparing models, and ensuring accuracy and up-to-date models.
AI-optimized Hardware:
Graphics processing units (GPU) and appliances specifically designed for AI-oriented computational jobs, make a difference in deep learning applications.
AI-optimized hardware refers to specialized hardware components and systems designed specifically to enhance the performance and efficiency of artificial intelligence (AI) workloads. These hardware solutions are tailored to meet the unique processing requirements of AI tasks, offering specialized architectures, parallel processing capabilities, and optimization methodologies to execute AI activities more quickly, efficiently, and at scale.
Key characteristics and benefits of AI-optimized hardware include:
-
Improved performance and real-time decision-making in AI systems.
-
Enhanced processing power and energy efficiency for AI applications.
-
Specialized designs and components to meet the computing needs of AI workloads.
-
Incremental improvements in user experience through real-time adaptation and optimization based on user input and data.
-
Efficient resource utilization, reduced computational waste, and optimized energy consumption in machine learning applications.
Decision Management:
Engines that insert rules and logic into AI systems, assisting in automated decision-making in a wide variety of enterprise applications.
Decision management is a systematic and data-driven approach to making and executing decisions within an organization. It involves the use of technology, data analysis, and business rules to streamline and improve decision-making processes.
Key factors for effective decision management include data quality, decision logic, automation, and adaptability. The three types of decision-making in management are operational, tactical, and strategic. Operational decisions are routine and day-to-day, tactical decisions focus on achieving specific objectives, while strategic decisions impact the organization's long-term goals.
Deep Learning Platforms:
These platforms consist of artificial neural networks with multiple abstraction layers, primarily used in pattern recognition and classification applications supported by large datasets.
These platforms provide a range of features and capabilities to support the development, training, deployment, and monitoring of machine learning models, catering to diverse user needs and preferences in the field of deep learning.
Biometrics:
Biometric technologies enable natural interactions between humans and machines, including image and touch recognition, speech, and body language, primarily used in market research.
Biometrics refers to the measurement and statistical analysis of people's unique physical and behavioral characteristics, used for identification and access control. It involves the use of biological or physiological attributes like fingerprints, facial recognition, voice patterns, iris recognition, and palm/vein patterns, as well as behavioral characteristics such as typing patterns, mouse movements, and gait, to verify an individual's identity.
Biometric authentication is increasingly common in security systems, consumer electronics, and point-of-sale applications, offering a more secure and convenient alternative to traditional passwords or security tokens. Biometric data is stored and compared using specialized devices and software, with components including a reader or scanning device, software for data conversion and comparison, and a secure database for storing biometric data.
While biometrics provide enhanced security and convenience, there are also concerns regarding privacy, potential hacking risks, and the need for additional protections like multi-factor authentication to safeguard biometric data effectively.
These specific AI technologies showcase the diverse applications and successful implementations of artificial intelligence in various organizations and industries.
Examples of successful AI
Some examples of successful applications of Artificial Intelligence include:
Maps and Navigation:
AI has significantly improved traveling experiences by providing optimal routes, real-time traffic updates, and enhanced visuals on maps.
Healthcare:
AI is revolutionizing healthcare with applications like disease prediction, drug development, personalized treatment recommendations, and robotic surgeries, leading to faster and more accurate diagnoses and improved patient care.
Finance:
AI is used in finance for fraud detection, algorithmic trading, credit scoring, risk assessment, and compliance automation, enhancing decision-making processes and improving efficiency in the financial sector.
Manufacturing:
AI applications in manufacturing include quality control, predictive maintenance, supply chain optimization, robotics, and smart manufacturing, leading to increased productivity, efficiency, and cost-effectiveness in production processes.
These examples showcase the diverse and impactful ways in which Artificial Intelligence is successfully utilized across various industries to enhance operations, improve outcomes, and drive innovation.
What's Your Reaction?
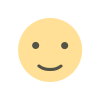
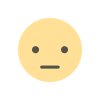
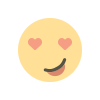
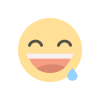
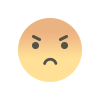
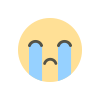
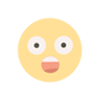