Utilizing Predictive Analytics in Mental Health EMR Software
Predictive analytics leverages historical data, machine learning, and statistical algorithms to predict future events and trends. When applied to mental health EMR software, it offers transformative potential.
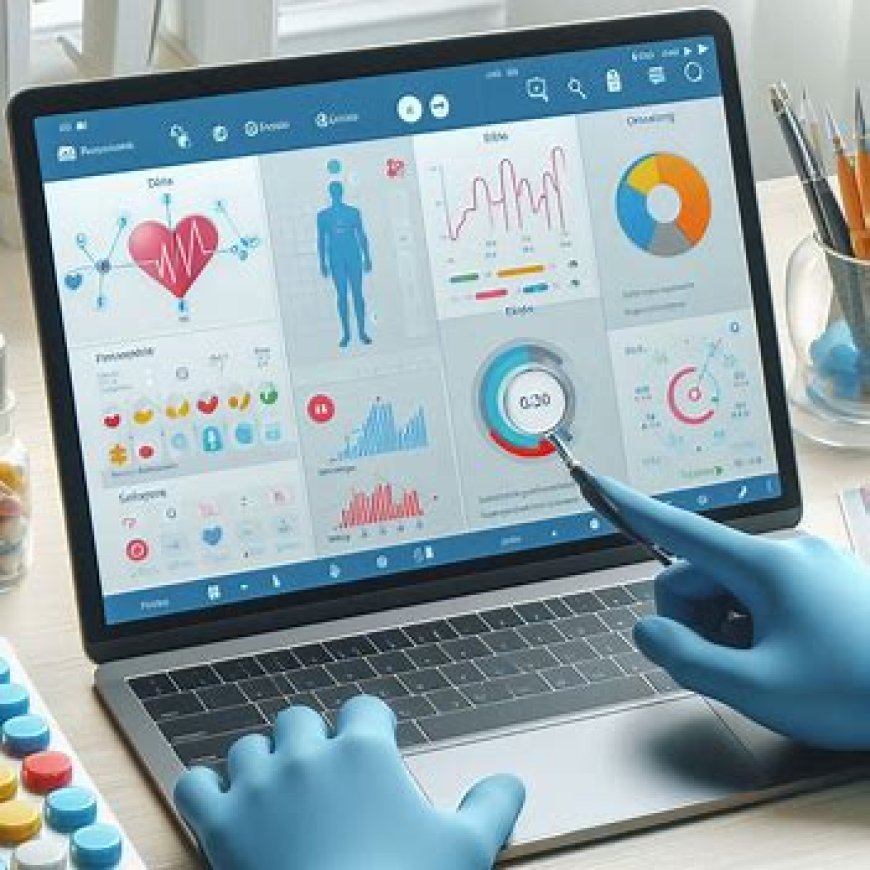
Introduction
The future of mental health care is here, and it is one of rapid change—fueled in no small part by technological innovation, holding out the promise of improvement in the quality and efficiency of patient care. One such innovation is the integration of predictive analytics in electronic medical record software. Predictive analytics is a technique of using historical data, machine learning, and statistical algorithms to predict future events and trends. When applied to mental health EMR software, it holds revolutionary potential in everything from early diagnosis to personalized treatment plans.
The Role of Predictive Analytics in Mental Health
Predictive analytics in mental health involves the analysis of large data volumes related to patients to establish trends that foresee the outcome. The data may originate from medical history, gene expression, life patterns, and even social determinants of health. In that respect, recognition of those trends and patterns gives better decision-making to healthcare providers hence better patient outcomes.
Benefits of Predictive Analytics in Mental Health EMR Software
Early Diagnosis and Intervention
- Early Detection: These predictive models can pick up early symptoms of mental health disorders, thus allowing for timely interventions before the conditions deteriorate.
- Risk Assessment: Based on the analysis of patient data, an EMR system can attach a risk to a possible mental health disorder and inform the clinician to pay more attention to those patients who require early treatment.
Individualized Treatment Plans
- Customized Therapies: Predictive analytics may be used to alter treatment plans concerning the individual profile of each patient to increase the chances of success.
- Medication Management: This would be the ability whereby, through an analysis of responses to past treatments, an EMR system proposes the best medication options for the patient, minimizing trial-and-error medication prescription.
Increased Patient Engagement
- Targeted Communication: Predictive models can flag those patients who may be on the verge of dropping out or otherwise becoming non-compliant so targeted communication and support can occur.
- Monitoring Outcomes: In such a way that, through the constant analysis of patient data, treatment progress is tracked and interventions adjusted accordingly, thereby keeping patients engaged and motivated.
Operational Efficiency
- Resource Allocation: By using predictive analytics, one can enable the optimum allocation of resources, such as staffing and appointment scheduling, against the predicted volume of patients that may be seen.
- Cost Reduction: It is expected to reduce costs by avoiding severe mental health crises and their associated treatment costs through early interventions.
Challenges and Considerations
Notwithstanding these significant benefits, there are challenges to the integration of predictive analytics into mental health EMR software, including:
Data Privacy and Security
- Sensitive Information: Mental health information is very sensitive, and thus it deserves the strictest security measures which must be carried out to protect the privacy of the patients.
- Regulatory Compliance: Regulations of HIPAA should be complied with to avoid litigation and other dire consequences which may shatter the patients' trust.
Data Quality and Integration
- Accurate Data: Predictive analytics' accuracy depends upon the data quality and completeness. It leads to incorrect predictions with incomplete or biased data.
- Interoperability: Predictive analytics, for effective implementation, would have seamless integration with existing EMR systems and other technologies in healthcare.
Ethical Considerations
- Bias and Fairness: Predictive models should be designed to avoid biases, especially those that may lead to unfair treatment or discrimination.
- Transparency: A set of guidelines on the transparency of the process regarding how predictions are being made and used will help retain trust among patients and clinicians.
Future Directions
The future of predictive analytics in mental health EMR software is bright provided that artificial intelligence and machine learning continue to develop. Some of the future predictions in this respect can be related to:
Better predictive models
- AI Integration: State-of-the-art AI techniques can provide better accuracy and reliability in the prediction models.
- Real-time Analytics: Inclusion of real-time data analysis for insights in real-time, increasing the responsiveness in mental healthcare.
Rich Sources of Data
- Wearable Technology: Integration of data from wearable devices can allow for the constant monitoring of patient's health and thus provide valuable insights into mental health trends.
- Social Media and Behavioral Data: Data extracted from social media and other behavioural sources may further provide context to mental health predictions.
Patient-Centered Care
- Empowered Patients: Patients, when provided with predictive insights, may take a more proactive role in managing their mental health.
- Collaborative Decision-Making: Greater engagement of patients in decision-making with providers could result in more tailored and effective treatment plans.
Conclusion
Predictive analytics thus becomes a quantum leap forward in the quest to improve mental health care. It helps health providers make better decisions because of the capacity to know and understand better out of the data, hence giving early interventions with personalized treatments that will lead to better patient outcomes. There still exist challenges, but the benefits potential that predictive analytics brings forward outweigh them to become a very compelling tool for the future of mental health care.
What's Your Reaction?
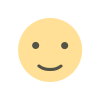
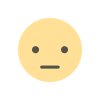
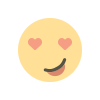
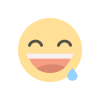
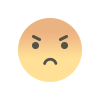
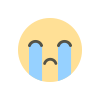
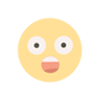