Choosing the Right Data Annotation Service Provider for Your Project
As a top Data labeling company, Objectways offers top generative ai, computer vision and NLP data labeling services. Contact us for accurate annotations.
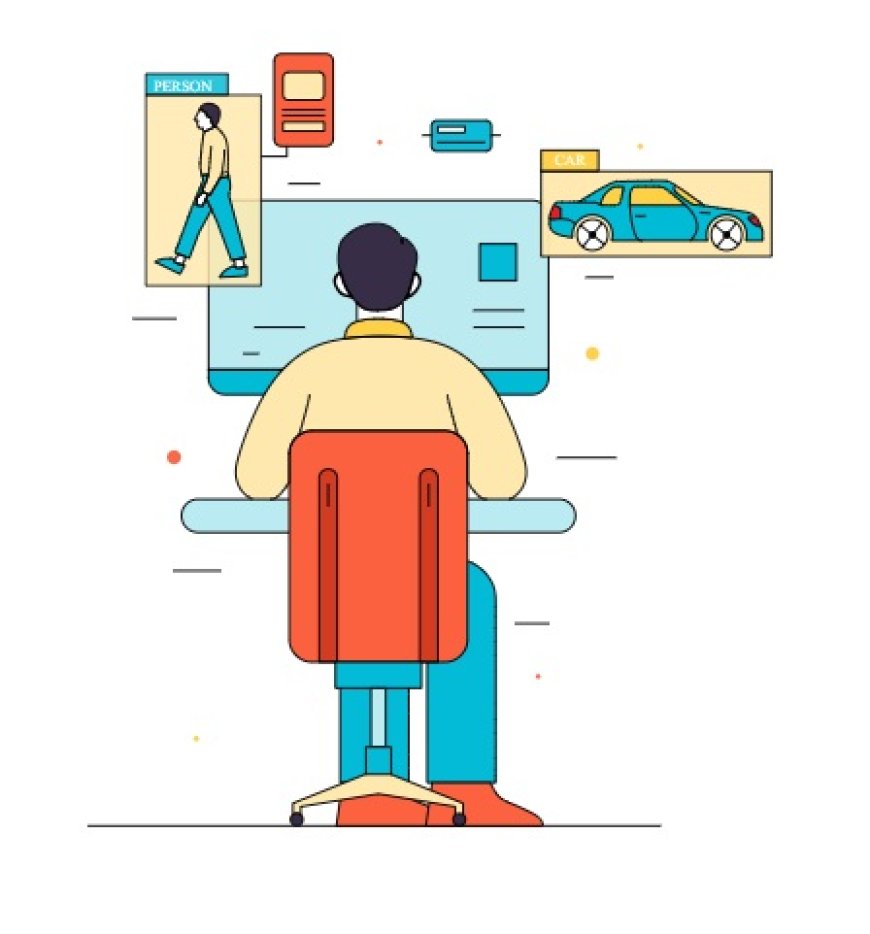
Are you ready to unlock the power of your data? In the digital age, harnessing the full potential of your datasets is crucial for driving innovation and making informed decisions. One key step in this process is data annotation – a vital task that requires precision and expertise. But how do you choose the right data annotation service provider for your project? Let's dive into the world of data annotation services together and explore what factors to consider, types of services available, best practices, top providers in the market, successful case studies, and more!
Understanding the Importance of Data Annotation
Data annotation is the process of labeling and categorizing data to make it understandable for machines. It provides the necessary context for algorithms to learn and improve accuracy. Without proper annotation, raw data remains unstructured and unusable. Imagine trying to teach a computer vision system without labeled images – chaos would ensue! Data annotation lays the foundation for machine learning models by guiding them on what to look for in datasets. This crucial step ensures that AI systems can recognize patterns, objects, or sentiments accurately in various applications. From self-driving cars to medical diagnostics, accurate data annotation is essential for reliable outcomes. The quality of annotations directly impacts the performance and reliability of AI systems, making it a critical aspect of any machine learning project.
Factors to Consider When Choosing a Data Annotation Service Provider
When selecting a data annotation services for your project, there are several key factors to keep in mind. Consider the experience and expertise of the company. Look for providers with a proven track record in delivering accurate and high-quality annotations across various industries.
Another important factor is scalability. Ensure that the service provider can handle your project's volume requirements efficiently without compromising on quality. Additionally, look into their annotation tools and technology to see if they align with your project needs.
Cost is also a significant consideration. Compare pricing structures among different providers to find one that offers competitive rates without sacrificing quality. Communication and support are vital aspects as well; choose a provider that maintains clear communication channels and provides responsive customer support throughout the annotation process.
Don't forget about data security measures implemented by the service provider to safeguard sensitive information during annotation tasks.
Types of Data Annotation Services Available
When it comes to data annotation services, there is a wide range of options available to cater to diverse project needs. One common type is image annotation, where objects within images are labeled for machine learning models to recognize patterns. Text annotation involves tagging and labeling text data for sentiment analysis or entity recognition tasks.
Another popular service is video annotation, which involves labeling frames in videos for object detection or action recognition purposes. Audio annotation focuses on transcribing audio files and annotating sound signals for speech recognition algorithms.
For more specialized projects, 3D point cloud annotation can be used in autonomous driving applications or robotics. Time series data annotation is crucial for forecasting and anomaly detection tasks in industries like finance or healthcare.
Each type of data annotation service offers unique benefits and plays a vital role in training AI models effectively. By understanding the different options available, businesses can choose the most suitable service provider for their specific requirements.
Best Practices for Working with a Data Annotation Service Provider
When working with a data annotation service provider, communication is key. Clearly define your project requirements and expectations upfront to avoid misunderstandings down the line. Be open to providing feedback and clarifications when necessary to ensure accuracy in the annotated data.
Establishing a timeline for deliverables is crucial for both parties. Set realistic deadlines and milestones to keep the project on track. Regular check-ins can help address any issues or updates that may arise during the annotation process.
Maintain transparency throughout the collaboration by sharing relevant information about your goals and objectives. This will help the service provider tailor their annotations to meet your specific needs effectively.
Prioritize quality control measures to ensure that the annotated data meets your standards. Conducting regular quality checks and audits can help identify any discrepancies early on, saving time and resources in the long run.
Top Data Annotation Service Providers in the Market
When it comes to choosing the right data annotation service provider for your project, there are several top players in the market that stand out for their quality and reliability. One such leading provider is Appen, known for its global crowd of annotators and expertise in various industries. Another top contender is Scale AI, offering high-quality training data for machine learning models with a focus on scalability and accuracy.
Labelbox is also a prominent player in the field, providing an intuitive platform for data annotation and collaboration among team members. Cogito specializes in human-in-the-loop services, combining AI technology with human intelligence to deliver precise annotations. Amazon Mechanical Turk offers a crowdsourcing platform that allows businesses to access a diverse pool of workers for efficient data labeling tasks.
Each of these top data annotation company brings unique strengths to the table, making them valuable partners for projects requiring accurate and reliable annotated datasets.
Case Studies: Successful Projects with Data Annotation Service Providers
In the realm of data annotation services, case studies serve as valuable examples of successful projects. One such instance involves a retail company that utilized image annotation to enhance its product recognition software. By partnering with a data annotation service provider, the company achieved higher accuracy in identifying products and improving customer shopping experiences.
Another compelling case study revolves around a healthcare institution that leveraged natural language processing annotation for medical records. The collaboration with a data annotation service provider resulted in more efficient analysis of patient information, leading to better treatment outcomes and streamlined operations.
Furthermore, an autonomous vehicle company saw significant advancements through sensor fusion annotation provided by an experienced data labeling partner. This enabled the vehicles to better interpret surroundings and make informed decisions on navigation routes, enhancing overall safety on the roads.
These success stories underscore the pivotal role data annotation service providers play in driving innovation across various industries.
Conclusion
Choosing the right data annotation service provider for your project is crucial for ensuring the accuracy and reliability of your machine learning models. By understanding the importance of data annotation, considering key factors when selecting a provider, exploring different types of services available, and following best practices in collaboration, you can set your project up for success.
With top data annotation service providers in the market offering specialized expertise and proven track records, you have a range of options to choose from based on your specific needs. By examining successful case studies that demonstrate the impact of partnering with data annotation experts, you can gain valuable insights into how these services can elevate your projects.
By making informed decisions and leveraging the expertise of reputable data annotation service providers, you can enhance the quality of your datasets and drive better outcomes in machine learning development. Remember to prioritize transparency, communication, and collaboration throughout the process to maximize the benefits of working with a trusted partner in data annotation services.
What's Your Reaction?
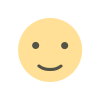
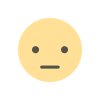
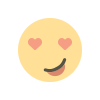
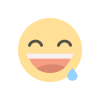
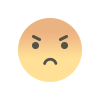
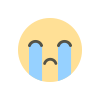
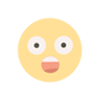