Scaling Up: Strategies for Managing Large-Scale Data Annotation Projects
As a top Data labeling company, Objectways offers top generative ai, computer vision and NLP data labeling services. Contact us for accurate annotations.
Introduction to Data Annotation and Its Importance
Welcome to the world of data annotation services, where every pixel, sentence, and image holds the power to transform industries. As businesses increasingly rely on machine learning algorithms and AI technologies to gain insights from vast amounts of data, the need for accurately annotated datasets has never been more crucial. However, managing large-scale data annotation projects comes with its own set of challenges and complexities that require strategic planning and innovative solutions for success. Let's dive into the realm of scaling up data annotation projects and explore effective strategies to navigate this dynamic landscape.
Challenges in Managing Large-Scale Data Annotation Projects
Large-scale data annotation projects come with their fair share of challenges that can make even the most seasoned project managers break a sweat. One major hurdle is the sheer volume of data that needs to be annotated accurately and efficiently within tight deadlines.
Keeping track of progress and ensuring consistency across annotations can be tricky, especially when working with a large team of annotators spread out geographically. Communication breakdowns and discrepancies in understanding annotation guidelines can easily lead to errors and inconsistencies in the labeled data.
Another challenge lies in maintaining quality control throughout the project lifecycle. With numerous annotators involved, ensuring uniformity in labeling standards becomes paramount to avoid skewed results or biased training data for machine learning models.
Moreover, scalability issues often arise when trying to expand operations rapidly to meet increasing demands for annotated data while balancing cost-efficiency. Finding ways to scale resources effectively without compromising on quality is a delicate balancing act that requires strategic planning and foresight.
Strategies for Effective Project Planning and Management
When it comes to managing large-scale data annotation projects, effective project planning and management strategies are key to success. One important strategy is setting clear goals and timelines right from the start. This helps keep the project on track and ensures that deadlines are met.
Another crucial aspect of effective project planning is breaking down the annotation task into smaller, manageable chunks. By dividing the workload into smaller segments, it becomes easier to assign tasks, monitor progress, and make adjustments as needed.
Communication is also vital in project management. Establishing regular check-ins with team members can help address any issues or concerns promptly. Additionally, leveraging collaboration tools such as project management software can streamline communication and enhance productivity.
Moreover, flexibility is essential when managing large-scale data annotation projects. Being adaptable to changes in priorities or requirements can help navigate unforeseen challenges and ensure successful project completion.
By implementing these strategies for effective project planning and management, organizations can optimize their data annotation processes and achieve high-quality results efficiently.
Utilizing Technology and Automation in Data Annotation
Technology and automation play a crucial role in streamlining large-scale data annotation projects. By leveraging advanced tools and software, the annotation process can be significantly expedited and made more efficient.
Automated algorithms can help in pre-labeling data, reducing the manual workload for annotators. This not only saves time but also improves overall accuracy by minimizing human error.
Furthermore, technology enables real-time collaboration among team members regardless of their locations, facilitating seamless communication and project management. Cloud-based platforms allow for secure storage and easy access to annotated datasets.
Machine learning models can be utilized to continuously improve annotation quality and consistency over time through feedback loops. This helps in maintaining high standards even as the project scales up.
Incorporating technology and automation into data annotation processes is essential for managing large volumes of data effectively while ensuring precision and timeliness in deliverables.
Best Practices for Quality Control and Assurance
Ensuring quality control and assurance is crucial in large-scale data annotation projects. One best practice is to establish clear guidelines and standards for annotators to follow. Providing detailed instructions and examples can help maintain consistency across the annotations.
Regularly conducting audits and spot checks on the annotated data can help identify errors or inconsistencies early on. Implementing a feedback loop where annotators receive constructive feedback can also improve the overall quality of annotations.
Utilizing tools like annotation validation software can automate error detection, making it easier to flag inaccuracies in real-time. Collaborating with domain experts or conducting inter-annotator agreement tests can further enhance the accuracy of annotations.
Prioritizing ongoing training for annotators on new guidelines, techniques, and tools is essential for maintaining high-quality standards throughout the project duration. By continuously monitoring and refining quality control processes, you can ensure that your data annotations meet the required level of accuracy and reliability.
Training and Motivating a Team of Annotators
Training and motivating a team of annotators is crucial for the success of large-scale data annotation services projects. Providing comprehensive training sessions on annotation guidelines, tools, and quality standards is essential to ensure consistency across annotations. Regular feedback and coaching help in improving annotator performance and addressing any issues promptly.
Creating a positive work environment with open communication channels encourages team collaboration and boosts morale. Recognizing and rewarding top performers can motivate the team to maintain high-quality output consistently. Implementing gamification elements like leaderboards or incentives adds an element of fun and competition which can drive productivity.
Investing in continuous learning opportunities such as workshops or skill development programs keeps annotators engaged and invested in their professional growth. Encouraging a sense of ownership over their work instills pride in their contributions to the project's success. Effective training coupled with ongoing motivation strategies fosters a dedicated team committed to delivering accurate annotations efficiently.
Cost Considerations for Large-Scale Data Annotation Projects
Managing large-scale data annotation projects involves careful consideration of costs to ensure efficiency and effectiveness. One key factor to bear in mind is the balance between quality and budget constraints. Choosing cost-effective annotation tools and platforms can help optimize resources without compromising on data accuracy.
Another aspect to consider is the scalability of the project, as costs may vary depending on the volume of data to be annotated. It's essential to assess the long-term financial implications and plan accordingly to avoid unexpected expenses down the line.
Additionally, implementing quality control measures from the outset can help prevent costly errors that may require rework or corrections later on. Investing in training for annotators and regular performance evaluations can contribute to overall cost savings by maintaining high standards throughout the project duration.
By carefully analyzing costs upfront, setting a clear budget framework, and continuously monitoring expenses, organizations can successfully navigate large-scale data annotation projects while staying within financial parameters.
Case Studies: Successful Examples of Scaling Up Data Annotation
In the realm of large-scale data annotation projects, success stories serve as beacons of inspiration. Let's delve into a few captivating case studies where scaling up data annotation has led to remarkable outcomes.
One prominent example is Company X, which leveraged advanced AI algorithms to streamline their annotation process. By incorporating smart automation tools, they significantly increased efficiency and accuracy in labeling vast datasets.
Another fascinating case study involves Research Institute Y, who implemented stringent quality control measures across their annotator team. Through continuous monitoring and feedback mechanisms, they achieved unparalleled levels of precision in their annotations.
Startup Z embraced a collaborative approach by fostering a culture of ongoing learning and development among their annotators. This investment in training resulted in a highly skilled workforce capable of tackling complex annotation tasks with finesse.
These success stories underscore the immense potential for growth and innovation within the realm of large-scale data annotation projects.
Conclusion: The Future of Large-Scale
As data continues to play a crucial role in various industries, the demand for accurate and scalable data annotation services will only increase. By implementing effective project planning, utilizing technology and automation, maintaining quality control measures, training annotators well, and managing costs efficiently, organizations can successfully manage large-scale data annotation projects.
The future of large-scale data annotation projects is promising as advancements in AI and machine learning continue to evolve. With the right strategies in place, businesses can harness the power of annotated data to drive insights, innovation, and competitive advantage in today's data-driven world. Embracing these best practices will ensure that organizations are well-equipped to handle the challenges and opportunities that come with managing vast amounts of annotated data effectively.
What's Your Reaction?
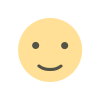
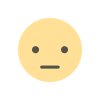
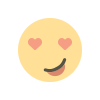
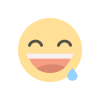
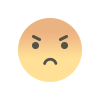
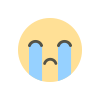
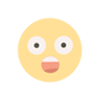