Maximizing Data Science Tools: 7 Strategies for Success
In the realm of data-driven decision-making, leveraging the right tools is essential for extracting valuable insights and driving impactful outcomes. Data science tools play a pivotal role in this process, enabling organizations to harness the power of data and gain a competitive edge in today's dynamic marketplace.
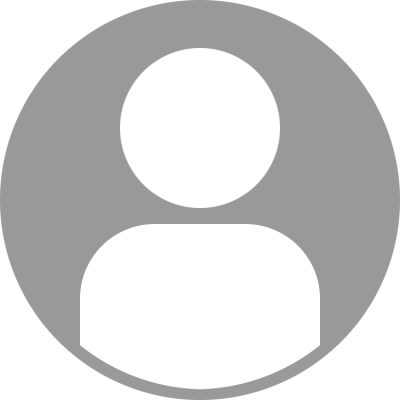