Data Analytics Tools and Software: A Comprehensive Overview
In today's data-driven world, organizations across industries are leveraging data analytics tools to extract valuable insights from their vast datasets. Data analytics involves the process of examining raw data to uncover patterns, trends, and correlations that can inform strategic decision-making and drive business growth. These tools play a pivotal role in transforming raw data into actionable insights, enabling organizations to gain a competitive edge and make informed decisions in real-time.
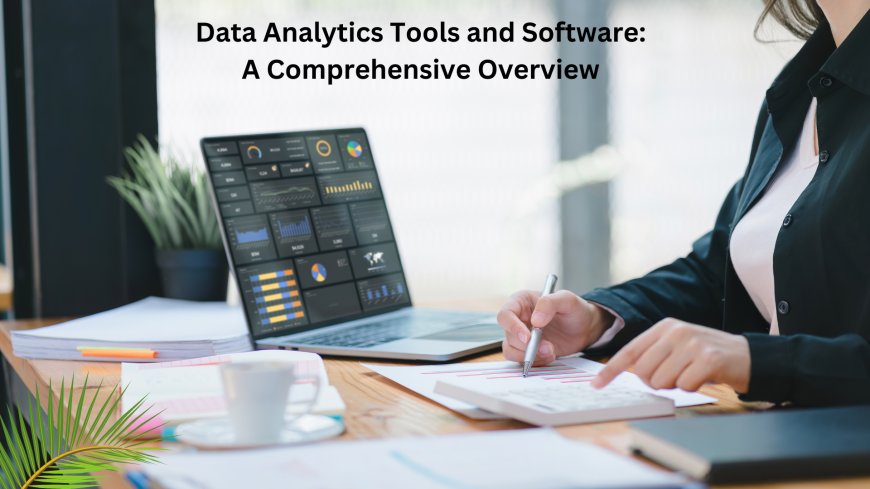
In today's data-driven world, organizations across industries are leveraging data analytics tools to extract valuable insights from their vast datasets. Data analytics involves the process of examining raw data to uncover patterns, trends, and correlations that can inform strategic decision-making and drive business growth. These tools play a pivotal role in transforming raw data into actionable insights, enabling organizations to gain a competitive edge and make informed decisions in real-time.
The landscape of data analytics tools is vast and diverse, encompassing a wide range of software applications, platforms, and technologies. From simple visualization tools to advanced machine learning algorithms, there is a tool available to suit every need and use case. These tools vary in complexity, functionality, and scalability, catering to the diverse needs of businesses ranging from startups to multinational corporations.
Types of Data Analytics Tools
Data analytics tools can be categorized into several types based on their functionality and the insights they provide:
- Descriptive Analytics Tools: Descriptive analytics tools focus on summarizing historical data to provide insights into past trends and patterns. These tools enable users to visualize data through charts, graphs, and dashboards, allowing them to gain a deeper understanding of historical performance and trends. Popular tools in this category include Microsoft Power BI, Tableau, Google Data Studio, and Excel.
- Diagnostic Analytics Tools: Diagnostic analytics tools are used to identify the root causes of issues and anomalies in data. These tools enable users to drill down into data sets to uncover insights and explanations for why certain events occurred. Diagnostic analytics tools often involve advanced querying and data exploration techniques, such as SQL-based querying tools and Python libraries like pandas and NumPy.
By leveraging these different types of data analytics tools, organizations can gain a comprehensive understanding of their data, uncover valuable insights, and drive informed decision-making. Whether it's visualizing historical trends, diagnosing performance issues, predicting future outcomes, or prescribing optimal actions, data analytics tools empower organizations to harness the full potential of their data and achieve their business objectives.
Descriptive Analytics Tools
Descriptive analytics tools serve as the foundational layer of data analysis, focusing on summarizing historical data to provide insights into past trends and patterns. These tools play a crucial role in transforming raw data into actionable insights by presenting it in a visually compelling and easily understandable format.
One of the key features of descriptive analytics tools is their ability to create visualizations such as charts, graphs, and dashboards. These visualizations allow users to explore data intuitively, identify trends, outliers, and correlations, and communicate insights effectively with stakeholders. Popular tools in this category include Microsoft Power BI, which offers a user-friendly interface and robust visualization capabilities, Tableau, known for its interactive dashboards and advanced analytics features, and Google Data Studio, a free tool that enables users to create customizable reports and dashboards using data from various sources.
Descriptive analytics tools are widely used across industries for a variety of purposes, including tracking key performance indicators (KPIs), monitoring business metrics, and analyzing customer behavior. By leveraging these tools, organizations can gain a comprehensive understanding of their historical data, identify areas for improvement, and make data-driven decisions to drive business success.
Diagnostic Analytics Tools
Diagnostic analytics tools build upon the foundation laid by descriptive analytics, focusing on identifying the root causes of issues and anomalies in data. These tools enable users to dig deeper into their data sets to uncover insights and explanations for why certain events occurred, helping them diagnose problems and take corrective actions.
One of the primary features of diagnostic analytics tools is their ability to perform advanced querying and data exploration. These tools allow users to analyze large volumes of data, apply filters and conditions, and drill down into specific subsets of data to identify patterns and anomalies. SQL-based querying tools, such as MySQL and PostgreSQL, are commonly used for this purpose, providing powerful capabilities for data manipulation and analysis.
Additionally, Python libraries like pandas and NumPy are widely used for data analysis and exploration, offering a rich set of functions and methods for manipulating and analyzing structured data. These libraries enable users to perform complex data transformations, calculate summary statistics, and visualize data using plots and charts, making them invaluable tools for diagnostic analytics.
By leveraging diagnostic analytics tools, organizations can gain deeper insights into their data, uncover hidden patterns and correlations, and identify opportunities for improvement. Whether it's diagnosing performance issues, detecting anomalies in customer behavior, or troubleshooting data quality issues, diagnostic analytics tools empower organizations to make informed decisions and drive continuous improvement.
Predictive Analytics Tools
Predictive analytics tools represent the next level of sophistication in data analysis, focusing on forecasting future trends and outcomes based on historical data. These tools leverage advanced statistical algorithms, machine learning techniques, and predictive modeling to identify patterns and relationships in data and make predictions about future events.
Machine learning platforms such as TensorFlow, scikit-learn, and Microsoft Azure Machine Learning Studio are popular choices for predictive analytics tasks. These platforms offer a wide range of algorithms and techniques for regression, classification, clustering, and more, enabling users to build and deploy predictive models with ease. Additionally, these platforms provide tools for data preprocessing, feature engineering, model evaluation, and deployment, streamlining the entire predictive analytics workflow.
Predictive analytics tools are widely used across industries for a variety of applications, including demand forecasting, customer segmentation, risk assessment, and fraud detection. By leveraging these tools, organizations can anticipate future trends and behaviors, identify opportunities and risks, and make proactive decisions to drive business success.
Prescriptive Analytics Tools
Prescriptive analytics tools represent the pinnacle of data analysis, focusing on recommending actions and decisions based on data insights. These tools go beyond descriptive and diagnostic analytics by not only explaining what happened and why but also prescribing optimal courses of action to achieve desired outcomes.
Prescriptive analytics tools encompass a wide range of techniques, including optimization, simulation, and decision support systems. Optimization tools, such as linear programming and integer programming solvers, are used to find the best solutions to complex problems with multiple constraints and objectives. Simulation software allows users to model and analyze different scenarios to understand their potential impact on outcomes. Decision support systems provide interactive interfaces and decision-making frameworks to help users evaluate options and make informed decisions.
One example of a prescriptive analytics tool is IBM Watson Analytics, which offers a suite of advanced analytics capabilities, including predictive modeling, optimization, and decision optimization. With Watson Analytics, users can analyze data, build predictive models, and generate actionable insights to inform decision-making and drive business outcomes.
By leveraging prescriptive analytics tools, organizations can optimize processes, allocate resources more effectively, and make better-informed decisions across a wide range of business functions. Whether it's optimizing supply chain operations, maximizing marketing ROI, or improving customer service, prescriptive analytics tools empower organizations to achieve their strategic objectives and stay ahead of the competition.
Conclusion
In conclusion, data analytics tools play a crucial role in helping organizations unlock the value of their data and gain actionable insights to drive business success. From descriptive analytics tools that summarize historical data to prescriptive analytics tools that recommend optimal actions, these tools enable organizations to make informed decisions and stay ahead of the competition in today's data-driven world. By leveraging a combination of descriptive, diagnostic, predictive, and prescriptive analytics tools, organizations can gain a comprehensive understanding of their data, identify opportunities and risks, and make data-driven decisions that drive business growth and innovation. Whether it's optimizing processes, forecasting future trends, or recommending strategic actions, data analytics tools empower organizations to harness the full potential of their data and achieve their business objectives. For individuals looking to enhance their skills in data analytics and gain hands-on experience with these powerful tools, enrolling in a Data Analytics Training Course in Noida, kochi, Nagpur, Delhi, etc, can provide valuable learning opportunities. By gaining practical knowledge and expertise under the guidance of experienced instructors, aspiring data analysts can develop the skills they need to excel in the field and pursue rewarding career opportunities in the rapidly growing field of data analytics.
What's Your Reaction?
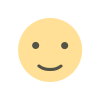
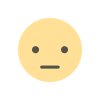
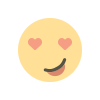
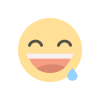
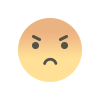
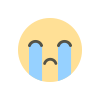
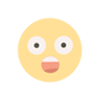