Mastering Data Wrangling and Cleaning: Essential Techniques for Aspiring Data Analysts in Nashik
Title: Data Wrangling and Cleaning Essentials in Nashik's Data Analyst Course In Nashik's data analyst course, students master techniques for handling missing data, outliers, and inconsistencies. They learn imputation methods, outlier detection, and data standardization for accurate analyses in real-world projects.
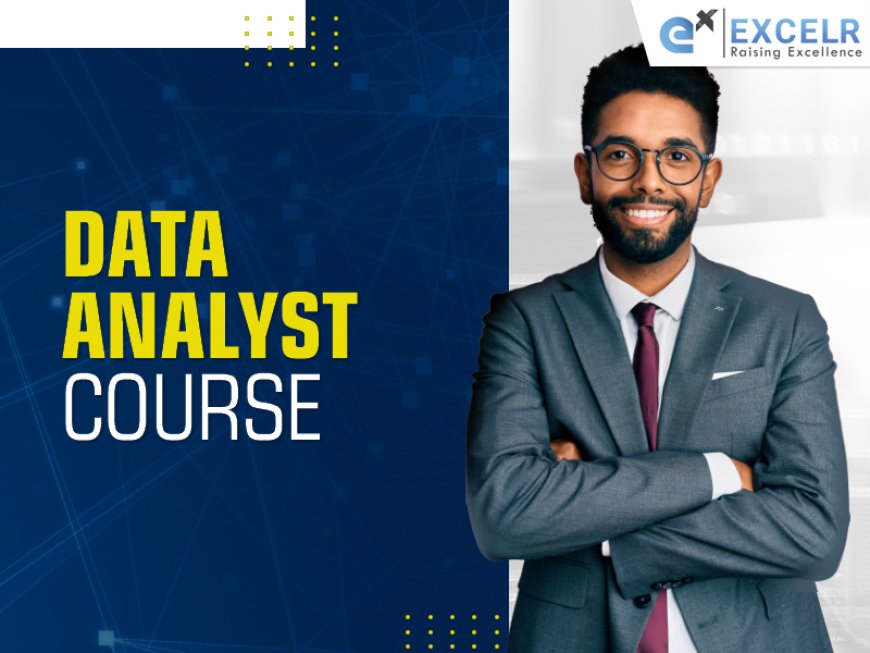
Introduction:
In the dynamic field of data analysis, the ability to wrangle and clean data is a foundational skill that can make or break the success of analytical endeavors. Aspiring data analysts in Nashik recognize the importance of mastering techniques for handling missing data, outliers, and inconsistencies in datasets. In this article, we delve into the essential aspects of data wrangling and cleaning within the context of a data analyst course in Nashik, exploring the tools and techniques that empower analysts to extract meaningful insights from raw data.
Understanding Data Quality Challenges:
Before diving into data wrangling and cleaning techniques, it's crucial for aspiring data analysts in Nashik to understand the common challenges associated with data quality. Datasets often contain missing values, outliers, duplicate entries, and inconsistencies, which can hinder the accuracy and reliability of analyses. In Nashik's data analyst courses, students learn to identify and address these challenges using a variety of techniques.
Handling Missing Data:
Missing data is a pervasive issue in real-world datasets and requires careful handling to ensure accurate analyses. In Nashik's data analyst courses, students are introduced to various strategies for dealing with missing data, including:
1. Imputation Techniques: Imputation involves filling in missing values with estimated or calculated values based on the available data. Students learn about methods such as mean imputation, median imputation, and forward/backward filling to address missing values effectively.
2. Deleting Missing Data: In some cases, it may be appropriate to remove observations with missing values from the dataset. Nashik's data analyst courses teach students how to assess the impact of missing data on analyses and make informed decisions about whether to delete or retain observations with missing values.
Handling Outliers:
Outliers are data points that deviate significantly from the rest of the dataset and can skew analytical results if left unaddressed. Nashik's data analyst courses equip students with techniques for identifying and handling outliers, including:
1. Visual Inspection: Students learn to use visualizations such as box plots, scatter plots, and histograms to identify outliers visually.
2. Statistical Methods: Nashik's data analyst courses introduce students to statistical techniques for detecting outliers, such as z-scores, quartiles, and the interquartile range (IQR).
3. Treatment Strategies: Depending on the nature of the data and the analysis objectives, students learn various strategies for handling outliers, such as transforming skewed data, winsorizing, or capping outliers.
Addressing Data Inconsistencies:
Data inconsistencies arise when different sources or data entry methods result in discrepancies within a dataset. Nashik's data analyst courses teach students how to identify and resolve data inconsistencies through techniques such as:
1. Standardization: Standardizing data formats and units ensures consistency across the dataset. Students learn to convert data into a standardized format to facilitate analysis and comparison.
2. Data Validation: Data validation involves checking data for accuracy and completeness. Students in Nashik's data analyst courses learn to use validation techniques such as range checks, format checks, and logic checks to identify inconsistencies and errors.
3. Data Cleaning Pipelines: Building data cleaning pipelines streamlines the process of identifying and correcting inconsistencies. Students learn to automate data cleaning tasks using tools like Python's pandas library or R's dplyr package.
Real-World Applications:
Throughout Nashik's data analyst courses, students apply data wrangling and cleaning techniques to real-world datasets from diverse industries such as healthcare, finance, retail, and agriculture. By working on hands-on projects and case studies, students gain practical experience in identifying data quality issues and implementing solutions to ensure accurate and reliable analyses.
Conclusion:
In conclusion, mastering data wrangling and cleaning techniques is essential for aspiring data analysts in Nashik to unlock the full potential of data and derive meaningful insights. By learning how to handle missing data, outliers, and inconsistencies, students in Nashik's data analyst courses acquire the skills needed to navigate the complexities of real-world datasets and make informed decisions based on reliable data. As Nashik continues to emerge as a hub for data analysis and innovation, the demand for skilled data analysts proficient in data wrangling and cleaning techniques is poised to grow, making it an exciting time to embark on a career in data analysis in Nashik.
What's Your Reaction?
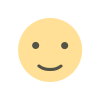
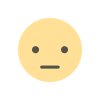
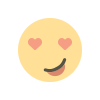
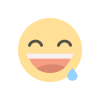
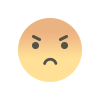
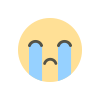
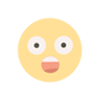